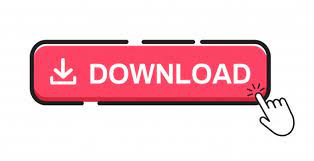
This is the most commonly used form of analytics among businesses. This type of analytics makes use of historical and present data to predict future events. These future incidents can be market trends, consumer trends, and many such market-related events. Predictive Analytics, as can be discerned from the name itself, is concerned with predicting future incidents. Taking the help of diagnostic analytics, the company comes out with a specific reason and then works on that to resolve the issue. The possible reasons behind this problem can be: the form didn't load correctly, the shipping charges are high, and not enough payment methods are available. Given the situation that the sales of the company have gone down even though customers are adding products to their carts. In business terms, diagnostic analytics is useful when you are researching the reasons leading churn indicators and usage trends among your most loyal customers.Ī use case for diagnostic analytics can be an e-commerce company. Techniques like drill-down, data mining, and data recovery, churn reason analysis, and customer health score analysis are all examples of diagnostic analytics. It gives a detailed and in-depth insight into the root cause of a problem.ĭata scientists turn to this analytics craving for the reason behind a particular happening. Recommended blog - Big Data in Manufacturingĭiagnostic Analytics, as the name suggests, gives a diagnosis to a problem. The company utilized its past data to increase its facility utilization across its offices and labs. This helps in creating reports like a company’s revenue, profits, sales, and so on.Įxamples of descriptive analytics include summary statistics, clustering, and association rules used in market basket analysis.Īn example of the use of descriptive analytics is the Dow Chemical Company. It simplifies the data and summarizes past data into a readable form.ĭescriptive analytics provide insights into what has occurred in the past and with the trends to dig into for more detail. This different approach of analytics gives rise to the four different types of Big data analytics.īig data analytics is categorized into four subcategories that are:ĭescriptive Analytics is considered a useful technique for uncovering patterns within a certain segment of customers. The different types of data require different approaches. This analytics tool is used by businesses to extract meaningful insights, such as hidden patterns, unknown correlations, market trends, and customer preferences, from a stack of raw and unstructured data. “Big data is high-volume, high-velocity, and/or high-variety information assets that demand cost-effective, innovative forms of information processing that enable enhanced insight, decision making, and process automation.”īig data analytics enables analysts, researchers, and business users to leverage big data, which was previously inaccessible and unusable, for faster and better decision-making. The database required to process big data should have low latency that traditional databases don’t have.īig data has one or more characteristics among high volume, high velocity, and high variety. We also reckon them as big data.īig Data is a term that is used for data sets whose size or type is beyond the capturing, managing, and processing ability of traditional rotational databases. These diverse data sets include structured, semi-structured, and unstructured data, from different sources, and in different sizes from terabytes to zettabytes. The process of analysis of large volumes of diverse data sets, using advanced analytic techniques is referred to as Big Data Analytics.

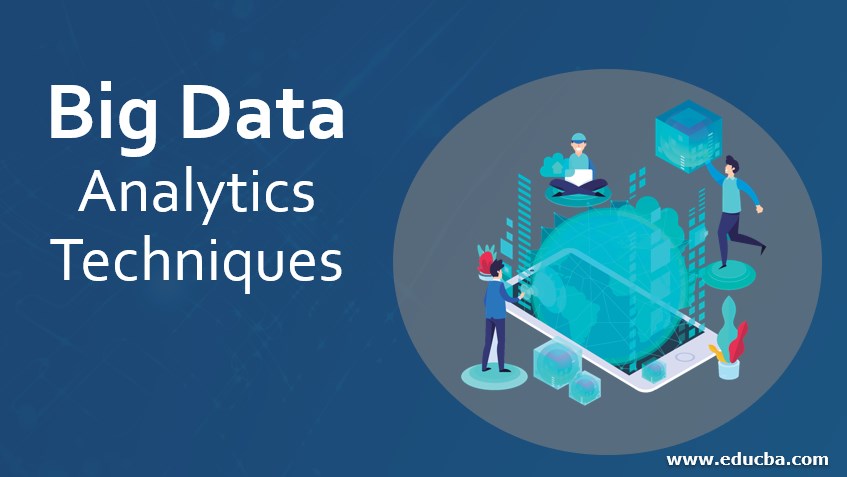
Through this blog, we will be exploring big data analytics, its different types, advantages of big data analytics, and its industrial applications. It has allowed businesses to know their customers better than they know themselves proving the technique to be extremely advantageous. The tools used for big data analytics have seen increased use in the recent past.īig data analytics has found several applications in different industries. Since traditional computing techniques cannot process these big data, various tools are being leveraged. The process of extracting meaningful insights from such raw big data is reckoned as big data analytics. We refer to these high volumes of data as big data.īusinesses, nowadays, rely heavily on big data to gain better knowledge about their customers. The high volumes of data sets, that a traditional computing tool cannot process, are being collected daily.

In an era where technology has reached the pinnacle of its use and has completely overpowered our lives, the amount of data exchanged is enormous.
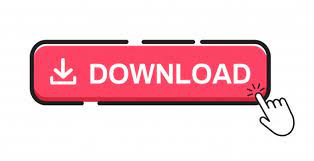